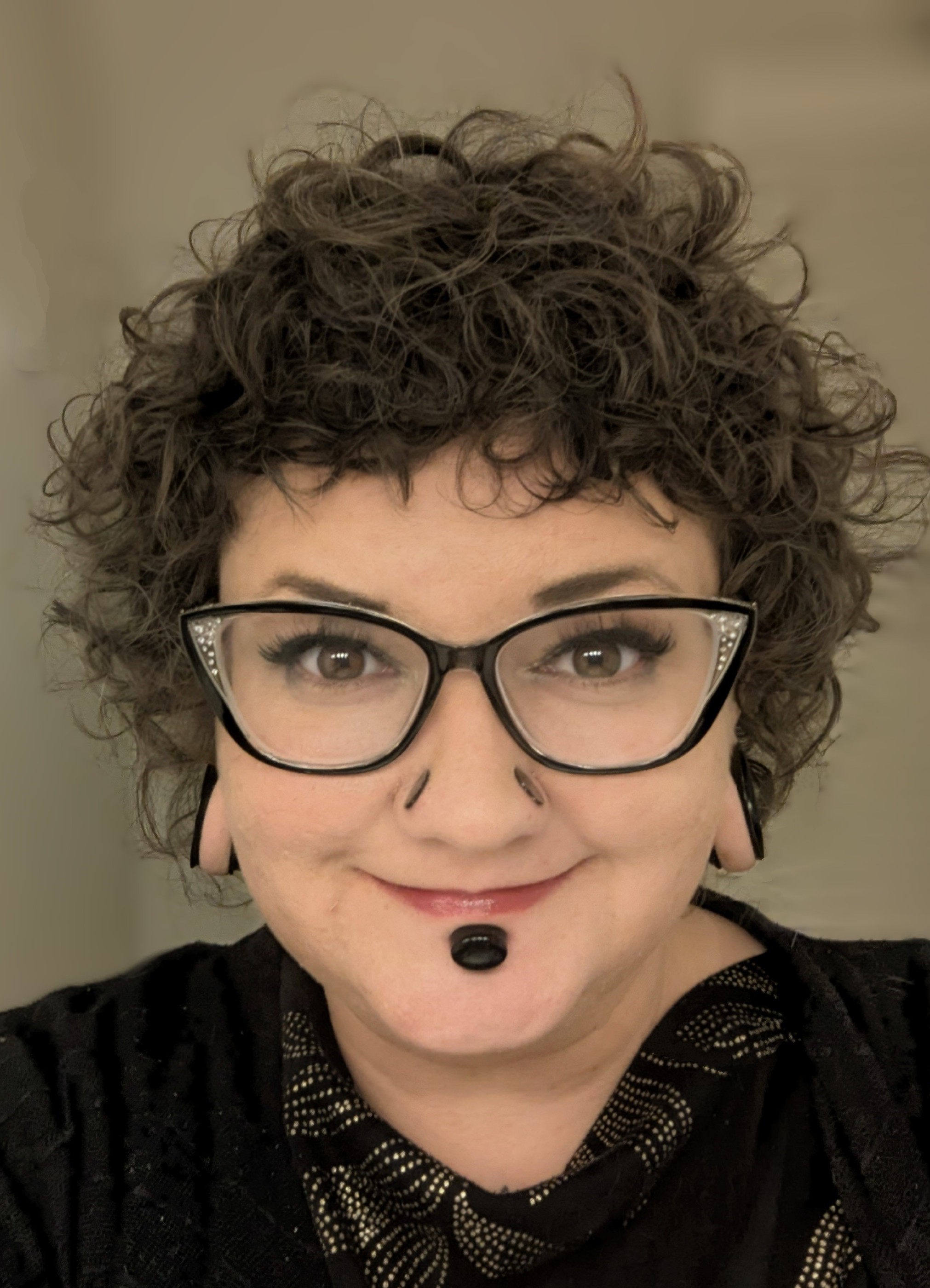
Lindley R. Slipetz
Quantitative Psychology PhD and Data Science MS student at University of Virginia
Ecological Momentary Assessment (EMA)
Psychological disorders are not static: they change over time, whether transitioning between healthy states and disordered states (e.g., from a healthy state to suicidal ideation) or transitioning within disordered states (e.g., from depression to mania). An overarching theme to my work is developing statistical tools to best model EMA data in a clinical setting. EMA data is typically measured multiple times a day over several days in the participant’s natural environment, and my interest lies in its promise in capturing the minutiae of the dynamics of psychological disorders. My main aim is to assess and develop new statistical methods and software for the analysis of EMA data that are practically applicable in the clinical context. This is evident in my research areas of specialization.
Preprint
Slipetz, L.R., Eberle, J., Levinson, C.A., Falk, A., Cusack, C., Henry, T.R. “Analyzing Ecological Momentary Assessment Data With State-Space Models: Considerations and Recommendations.”
Network Psychometrics
My interest in network psychometrics stems from its ability to capture dependencies among symptom variables and the emergent properties and topological structure that arise from these relations across time, potentially resulting in better diagnoses and treatments of mental disorders. Here my work focuses not only on clinical application, but, also, creating and testing methods with the goal of investigating the scope and limits of network methodology.
Publications
Levinson, C. A, Slipetz, L.R., Henry, T.R., Pennesi, JL., Crumby, E. “What Makes Personalized Treatment Work? Mechanisms of Change in Transdiagnostic Network-Informed Personalized Treatment for Eating Disorders.” Behavior Therapy (2025)
In Revision
Dynamical Systems
Much of my interest in dynamical systems comes from the context of state-space modeling, a general modeling framework with a broad reach and well-suited to handling the complexities of EMA studies, including missingness, time trends, and non-stationarity. A goal in this arena is to develop state-space modeling methods and software that can handle the heterogeneity of data types inherent in EMA and apply them to clinical problems. My dissertation utilizing regime switch forecast techniques to predict phase transitions in psychological disorders.
Publications
Slipetz, L.R. , Henry, T.R. “Dynamical Systems Approaches to Modeling Psychopathological Processes.” Oxford University Press Book Chapter (2025).
R package
Falk, A., Slipetz, L.R.,, Henry, T.R. (2023). netlabUVA/genss: genss v0.1.0 - Initial Prerelease (v0.1.0). Zenodo.https://doi.org/10.5281/zenodo.7887019
Dissertation
This project aims to utilize forecasting regime switching techniques to predict phase transitions into psychopathological disease states via the methodologies of quantitative and clinical psychology. Specifically, the applicant will develop these techniques based on previously developed models of financial volatility, making predictions about the development of psychopathology based on these models, evaluating practical data requirements, and applying the results to a real world case of developing psychosis.
Slipetz, L.R. (2027). “Predicting psychopathological phase transitions using forecasting techniques in regime switching models”
Generative AI and Large Language Models (LLMs)
A benefit of EMA data collection is that the large volume of data collected provides an informative basis for building psychopathological trajectories. A downside of EMA data collection is that the large volume of data is a hinderance to statistical analysis, particularly in the case of text data. We need methods of analyzing large volumes of EMA text and, to do this, we need large volumes of text to test our methodologies. In this arena, I have been working on text generation for use EMA methods development.